Emergency Medicine & AI: What Will Change in the Next 5 Years?
Apr 20, 2025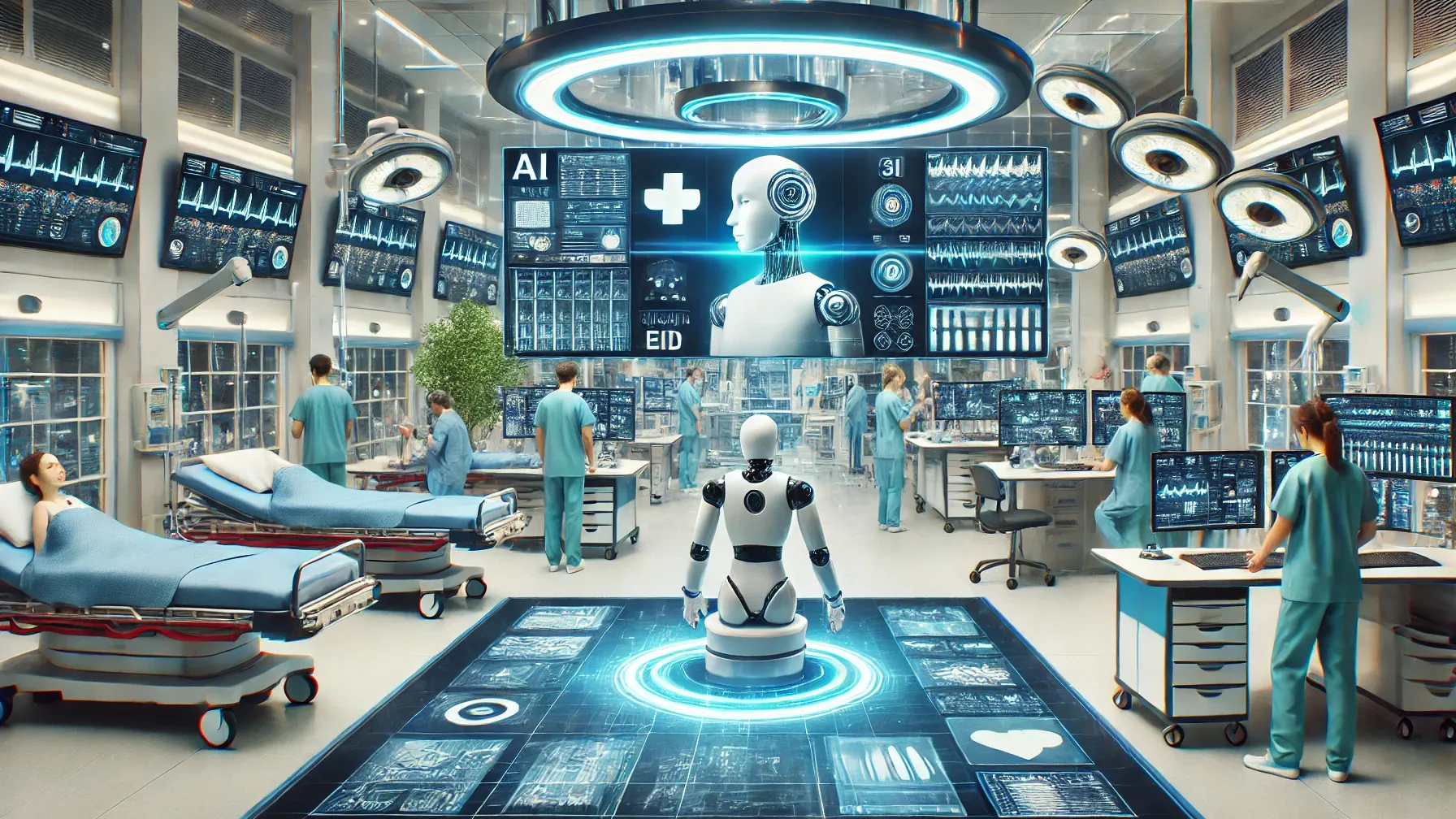
Introduction
In emergency medicine, we're used to buzzwords—“blockchain,” “virtual wards,” “integrated care pathways.” But one that’s sticking around for the long haul is artificial intelligence (AI).
The question isn’t whether AI will affect the ED. It’s how, when, and whether we’ll be ready.
Here’s a grounded look at how AI will realistically reshape emergency care within the next 5 years—no sci-fi hype, just practical examples, clinical relevance, and critical considerations.
1. AI-Supported Triage: The First Five Minutes Will Look Different
Current Problem:
Triage depends on human interpretation of risk. It's inconsistent between staff, shifts, and hospitals. Some patients with subtle warning signs are downgraded; others are over-prioritised.
What AI Will Change:
AI triage tools will not replace nurses. But they’ll run quietly in the background, pulling data from vitals, past attendances, and real-time health records to flag risk.
Real Case:
A 64-year-old woman presents with back pain. Normal vitals, stable pain. Triage scores her as Category 4 (non-urgent). But an AI tool notes she had a recent CT for pancreatic cyst follow-up, a raised D-dimer last week, and subtle hypotension on arrival (sBP 100). It flags the possibility of a leaking AAA.
Outcome: She gets early POCUS and is sent for urgent vascular referral. A life saved.
What's needed: Integration with national EPRs (e.g. NHS Spine), robust validation, and human override.
2. AI in Imaging: Radiology Bottlenecks Will Shrink
Current Problem:
CT head reports at night often arrive an hour later. Chest X-ray delays can bottleneck decision-making. Fractures and pneumothoraces are sometimes missed.
What AI Will Change:
AI will act as a real-time second reader, flagging urgent abnormalities even before the radiologist reviews them.
Real Case:
A 72-year-old man with AF presents post-fall. Head CT is done. Before the radiologist reads it, the AI system flags a subtle right-sided subdural. ED team gets a call on the alert.
Outcome: Early neuro referral, reversal of anticoagulation, transfer. Time saved: 45 minutes.
What's needed: Regulatory approval (MHRA/CE-marked software), agreed false positive thresholds, and staff training to avoid over-reliance.
3. Clinical Decision Support: AI Will Nudge, Not Dictate
Current Problem:
Junior doctors often lack experience with nuanced cases. Senior review can be delayed. Guidelines are long and often not embedded into real-time workflows.
What AI Will Change:
AI systems integrated with ED software (e.g. Symphony, Cerner) will provide context-aware nudges—like early sepsis alerts, risk of ACS, or PERC score recommendations—based on live data entry.
Real Case:
A 45-year-old man with pleuritic chest pain and a recent leg cast presents. D-dimer is borderline. He’s set for discharge. But the AI system highlights a high Wells Score based on coded symptoms and recent travel history.
Outcome: CTPA confirms a large PE.
What's needed: Transparent algorithms, clear explanation of why a prompt was generated, and human override.
4. Predictive Modelling: Smarter Resource Planning, Not Crystal Balls
Current Problem:
Emergency Departments face crowding, delayed admissions, and corridor care—not because we lack beds, but because we lack timely foresight into who will need them.
How AI May Help:
Live AI prediction models (already in testing across several UK Trusts) can forecast which patients are likely to be admitted within minutes of arrival—based on presenting complaint, age, triage, labs, and past history.
AI-powered predictive tools (already in pilot use across several NHS Trusts) can analyse real-time data—presenting complaint, triage category, bloods, comorbidities—to estimate the likelihood of admission within minutes of arrival.
These models do not decide who gets admitted. Instead, they offer early signals to inform staffing and bed planning.
Realistic Scenario:
During a winter surge, an AI system identifies that 7 patients in the waiting room have a high probability of requiring medical admission. The bed manager uses this forecast to open contingency capacity 90 minutes earlier than planned.
Outcome: Reduced delays, safer flow, and no patients left waiting on trolleys.
Caveats and Safeguards:
This isn’t magic. AI predictions must be:
- Regularly validated and adjusted to reflect local population changes
- Supplemented by clinical judgement, never replacing it
- Transparent in how predictions are made (avoiding “black box” tools)
Bottom Line: Predictive AI isn’t about certainty—it’s about better-informed decisions in a pressured system.
5. AI in Documentation: The End of the Typing Burden?
Current Problem:
ED clinicians spend 30–50% of their shift typing. Documentation fatigue leads to errors, burnout, and poor narrative quality.
What AI Will Change:
AI scribes (using voice recognition and natural language processing) will convert clinician-patient conversations into structured notes in real time—editable, but 90% complete.
Real Case:
In a high-acuity resus, the registrar speaks aloud during assessment (“GCS 12, right pupil fixed, systolic 85, chest clear”) while managing the airway.
Outcome: By the time the patient goes to CT, the history, exam, vitals, and interventions are already documented. Time saved: 15–20 minutes per patient.
What's needed: Data security, high-fidelity microphones, and fallback options for noisy environments.
Caution: What AI Won’t Do
Let’s be clear—AI won't:
-
Replace human intuition, compassion, or ethics.
-
Make final diagnoses.
-
Take responsibility for bad outcomes.
AI augments. It does not absolve.
Takeaway Messages
-
AI tools will support, not replace, frontline emergency clinicians.
-
Expect incremental change—triage flags, image pre-reads, nudges in documentation and disposition.
-
Clinical oversight remains paramount—with AI, the ultimate liability still rests with the doctor.
-
Prepare now: get involved in pilot programmes, learn how these tools work, and don’t be the last to adapt.
Final Thought
Emergency Medicine is chaotic, pressured, and time-critical. AI won't solve all its problems—but it might just buy us back the most precious resource we have: time to think.